Insurance companies are awash in an ocean of information, beset by new data streams and technologies. The problem? They don’t currently have the IT capacity to use this data to navigate their future. Machine learning can change that.
Celestial navigation and machine learning
Before the invention of sonar or GPS, people relied on celestial navigation — “dead reckoning” — to get from point A to point B. And before the internet, we had the yellow pages and the phone book. In the wake of every technological leap forward, it feels obvious, in retrospect, that information could and should have been organized more accurately and marshaled more effectively to our ends than it was. Compared with any major advancement, past technologies and methods seem as crude to us as dead reckoning.
Machine learning, also known as the ongoing revolution in artificial intelligence, represents just such a leap forward for several industries, chief among them the insurance industry. Insurers today face a stark choice: invest early in a technology that could dominate and shape the next 10 years in their field, or continue to try navigating by starlight and risk ending up stranded on the wrong shore entirely.
Making sense of all this data: machine learning
The area where machine learning shines brightest is in processing large amounts of often-repetitive data. Just look at Lemonade, which utilizes machine learning and chatbots in order to process and resolve claims in seconds. They’re not just flipping the claims process on its head—they’re using AI to marshal data that they already possess more effectively. But insurers don’t need to explode their entire business-model and begin life anew as a startup in order to take advantage of machine learning technology. Geico is applying AI to car and home videos, to automatically assess damage claims on the one hand, and estimate repair costs on the other. This isn’t just a neat idea for insurers going forward, it is a competitive necessity. The potential payoff for the insurance industry, if they do integrate the technology to pluck meaning from these massive data sets is commensurately huge, from risk reduction to pricing innovation and dollars saved to money earned.

There are three discrete areas where machine learning will likely have the biggest impact on insurance:
Making claims verification faster, smarter, and simpler
Lemonade and Geico have already provided real-world examples of how machine learning can very nearly automate the entire claims process from end to end. A given program can pull a digital file, analyze it via a pre-programmed algorithm, and automatically assess a claim in a very short time window, rather than the claim toiling in obscurity at the bottom of a backlog of other claims just like it. But it's not just about chatbots and algorithms. Companies like Snorkel for example, have identified that the biggest hurdle in implementing machine learning in insurance isn’t just in designing the perfect model. It’s more about sorting through a bunch of disparate and disconnected datasets, and automatically labelling them using a more complete set of classifications, heuristics and ontologies. That’s to say: building a more complicated machine isn’t necessarily the answer. Sort of like Occam’s razor: sometimes the answer is a simpler Machine Learning pipeline equipped with a more effective data model, rather than an over engineered one with an incomplete or ineffective data model. Ultimately your machine learning implementation will only be as good as the data model underlying it.
Automatically assessing and underwriting risk
Machine learning can be instrumental in assessing and flagging underwriting risks for insurers faster and more accurately than any individual crunching the numbers ever could. That isn’t to say that machine learning technology could or should wholly supplant human analysts in figuring out risk, but AI and machine learning technologies can flag liabilities that might have otherwise flown under the radar for a human analyst. by routing orders to different workflows based on underwriting risks, and by flagging factors that increase those risks in the first place, machine learning technologies make those sorts of scenarios far less likely and save insurers from having to make costly, avoidable payouts on bad policies.
If this sort of risk analysis is automated, then software can flag obvious warning signs for review long before a human analyst would see them. With a fully integrated machine learning risk assessment process, insurers can direct human resources where they will be most useful rather than wasting precious staff hours on the nuts and bolts of the review process. This sort of revolutionary thinking isn’t purely academic either. Companies like Alteryx are already helping insurers to calculate policy risk and predict future claims to optimize pricing, segment policy holders by profitability potential, and identify cross-sell targets in the process. If all that sounds incredibly dry, the upshot is anything but: machine learning cuts up and segments customer data faster and more accurately than ever before, and in the process turns a bunch of erstwhile liabilities into revenue generators virtually overnight.
Experience studies
By using machine learning to discover predictors in previous claims activity, insurers can then use that information to set future assumptions and feed them into pricing models and actuarial analysis. The fact is that machine learning software excels at picking up exactly the sorts of patterns that elude human analysts — either because we have a difficult time separating the signal from the noise or because the pattern itself is too faint for us to pick up on in the first place.
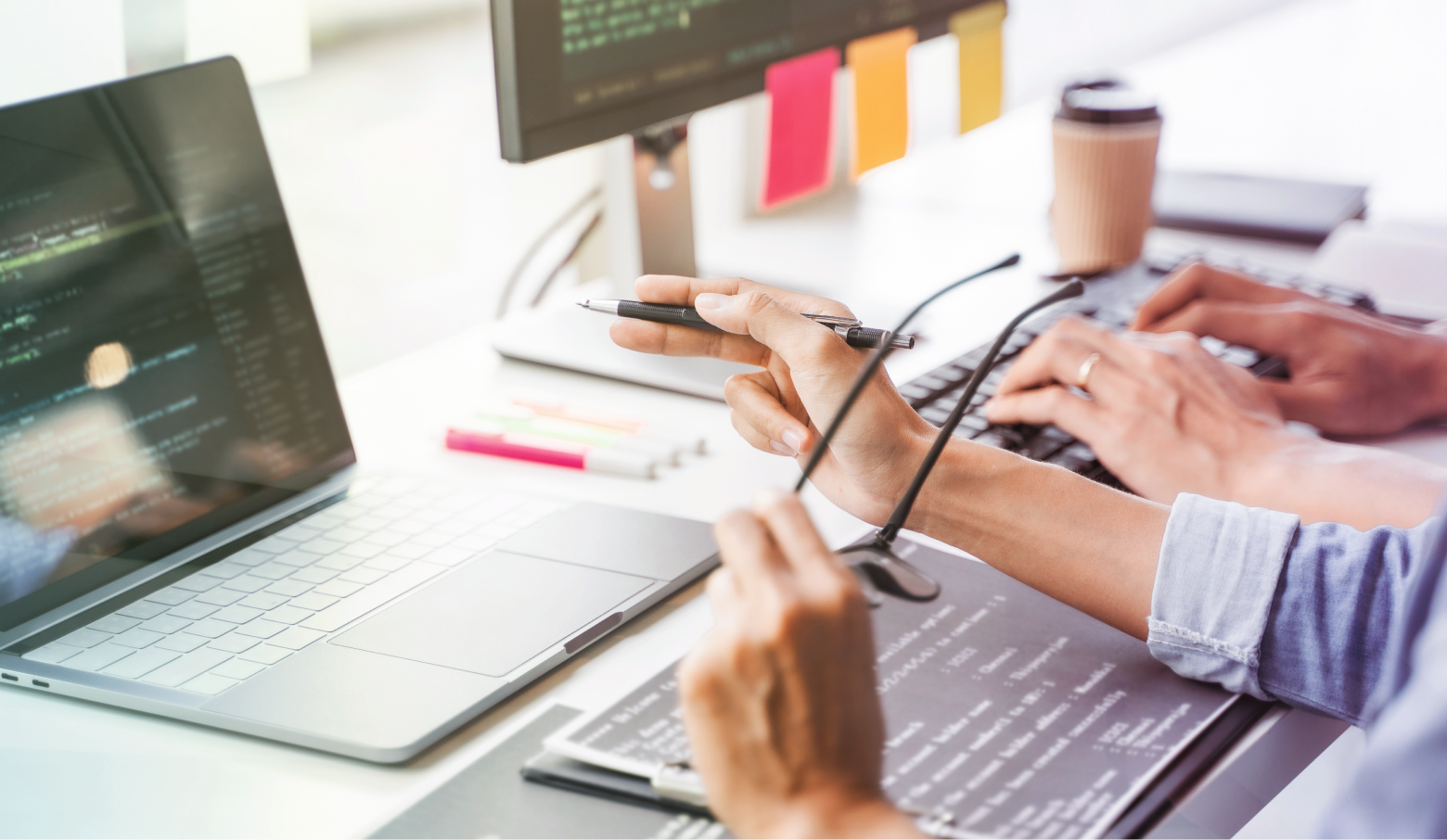
Awash in Data
Machine learning is a navigational tool, but it cannot work on its own. GPS coordinates don’t do much good if you can’t relay them to the captain or don’t have the ability to turn the ship in the first place. Machine learning has to be part of a comprehensive and integrated software apparatus that overhauls an insurer’s legacy network, enabling every person in every leg of the claims or assessment process to communicate easily, transparently, and with minimal redundancy. An integrated software network can streamline interactions between not just companies and clients but the whole gamut of individuals and specialists whose work goes into everything from actuarial assessments to claims adjustment.
Insurers now are swimming in more available information than they know what to do with. While human analysts and claims processors are vital parts of any successful insurance company, there’s an upper limit to how much data a single person can process at once. This is not a sink-or-swim moment, though. It is not like companies who don’t prioritize machine learning will suddenly be swallowed beneath an ocean of data. But it is an ocean. The question facing insurers is whether they’ll employ the tools available to navigate it or be stuck at sea while competitors race ahead.
Want to learn more about software integration? TheoremOne has teams of dedicated specialists available to answer questions about legacy modernization, machine learning and more. Reach out for a free consultation.