TheoremOne Labs holds the mission to evangelize the TheoremOne Way through the open exploration, experimentation, and iteration of ideas to share new technologies and ways of working. One such example is our TheoremOne Labs Smart Grid project which we used to explore machine learning and the energy sector.
As part of a two-pronged approach, we sought to learn how machine learning (ML) can help solve energy problems in the U.S. and created a proof of concept that predicts electricity usage for a U.S. state using a machine learning model.
TheoremOne Lab's Justin Kear explains how this project deepened our understanding of the advantages, challenges, and process of developing and deploying an ML model and the pain points faced by customers and energy companies.
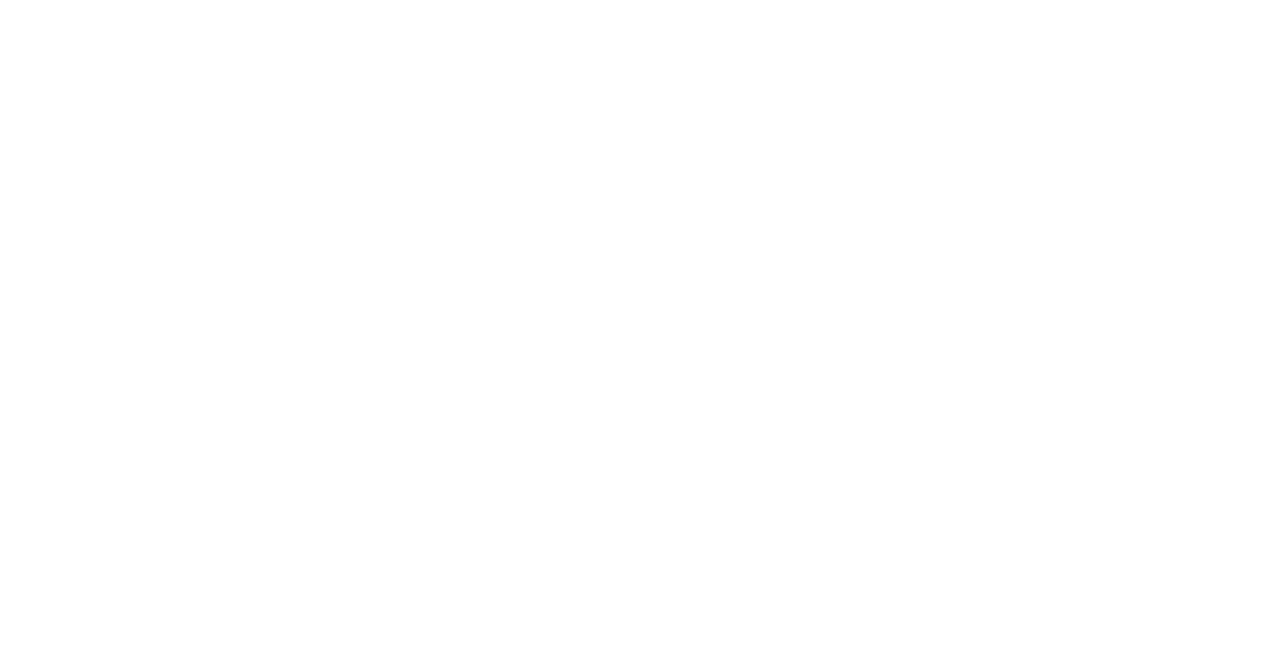
TheoremOne: What were the key objectives going into this project?
Justin: We wanted to define something tangible that we could build upon and wrap our heads around. The key focus of this Smart Grids Project is about bolstering our understanding of the energy sector and machine learning and addressing a particular machine learning problem.
TheoremOne: What is that machine learning problem?
Justin: We wanted to visualize energy usage and trends using selections of 15 years of historic electricity and weather data. We also wanted to test the performance of our machine learning model by asking it to predict electricity usage — specifically monthly energy usage by state. We expected utility companies, energy companies — both private and public — or even states to be interested in that electricity usage data to better plan.
TheoremOne: What kind of data did you use, and where did you get your data?
Justin: We pulled public sales and megawatt-hours for each state from the EIA (Energy Information Association). We also leveraged quite a bit of weather data as part of our machine learning model. We hypothesized that weather would be a key feature in the predictions since energy usage changes throughout the year, especially in different parts of the country. That's another area for iteration — energy usage in relationship to weather patterns and trends. We also looked at the time series data based on each month's weather data. All of this was plotted and diagrammed visually so that patterns and other features could be analyzed and understood, allowing us to continue to perfect the model.
ThereomOne: What were some of the biggest takeaways about machine learning you drew from this process?
Justin: It's all about the quality of the data you feed into the model. We learned that the smart grid specifically has two aspects — software and hardware.
- Software: the machine learning algorithms, the data, and the predictions.
- Hardware: the Internet of Things (IoT) smart sensors that collect the data.
These elements used in conjunction with one another solve the problem at hand.
TheoremOne: What problems and pain points exist in the energy sector, particularly those related to the smart grid?
Justin: Let me break the pain points down by customers and energy companies, as each group has different perspectives and challenges.
Customer Pain Points:
- They're concerned about choice, transparency, cost savings, and energy mix.
For example, customers want to know where their energy originates and choose where they get their energy. Many customers are interested in getting their power from renewable energy sources like solar instead of coal.
- They are also interested in gaining insight into their energy usage, particularly when it comes to increasing their household efficiency.
What's neat is that a smart grid allows for insights into usage metrics and opportunities for efficiencies. Similarly, customers are concerned about cost savings — they want to reduce their electricity bill and like the option to buy back energy that they don't use.
- The last piece that affects customers — and companies, to some degree — is the energy mix features, like being able to help establish what's the best way to set up solar panels and other alternative energy technology.
If you have solar panels in a particular area, customers and companies face problems deciding on proper angles, the number of panels needed, the number of wind turbines needed, placement, and other basic setup know-how. This helps companies establish solar or wind farms and assists individuals in their own homes.
Energy Company Pain Points:
- The key pain points we saw were reliability, stability, maintenance, disaster prevention, distribution, and infrastructure expansion.
There are three energy grids in the United States — the Western Grid, Eastern Grid, and Texas (ERCOT) Grid. As a significant energy producer, Texas has its own grid because it has numerous refineries and solar and wind farms throughout the state. But, in February 2021, the temperatures dropped to an abnormally low level for a week. As a result, the grid froze. Many Texans didn't have heat or electricity. They didn't have working refrigerators, nor could they cook. Even water filtration facilities weren't working correctly. Over that week, Texas experienced an energy emergency.
Typically, when something similar happens throughout the Eastern or Western grid service areas, they can pull from different backup sources. Since Texas is on its own, it didn't have any backups. So, this moment was a confluence of the reliability, stability, maintenance, distribution, and disaster prevention issues that energy companies worry about — all at once. From an energy perspective, preventing and predicting these emergencies is extremely useful.
- Companies care about infrastructure expansion.
Again, Texas has scorching summers. Sometimes in this season, Texas experiences brownouts where the grid is overtaxed, requiring them to manually shut down electricity to prevent overwhelming the grid. That comes down to the distribution of energy infrastructure and storage.
TheoremOne: So, the insights that the smart grid generates empower companies and individuals in their energy usage and disaster management??
Justin: Exactly. How cool would it be if you lived in Texas and you had some choice in where your electricity came from? If customers were offered more options due to these data insights, disasters like that in 2021 could be avoided.
The smart grid benefits the companies, too. Machine learning and data can power efficient usage, disaster avoidance, and prevention and help companies provide better or more desirable service to the customer.
TheoremOne: Sounds like a win-win. As we advance, what are the hopes with the project and with machine learning and energy?
Justin: It would be interesting to have data and machine learning algorithms leveraged to predict the demand and generation at a more precise level. We've also discussed using our machine learning model and comparing its outputs instead of the current way we predict energy usage to see if our model may be more accurate.
We see a lot of potential in getting more granular with the model — like what would energy usage look like in specific parts of each state or regions of the country? As I said before, that may impact the features we choose for the model and may give us insight into different aspects of energy usage.
Key Takeaway
Through the Theorem Labs Smart Grids project, Theorem Labs gained incredible insight into the world of machine learning, energy, and climate technology. We've found new ways of working with ML data and invented new ML processes, tools, and collaboration models — all of which are beneficial to any company looking to facilitate conversions around business growth through machine learning, energy, and ClimateTech.